Eric Horvitz, the Chief Scientific Officer at Microsoft, recently said: “We’re in the early days of a revolutionary era of digital biology rising at the convergence of biology and computer science. Advances at the intersection will fundamentally change our understandings about the mysteries of biology, with extraordinary implications for health, medicine, and sustainability.”
Such strong words make one wonder where this enthusiasm comes from. The answer lies in an emerging, but fast-accelerating, trend — the digitisation of biology. To understand why this is happening right now, we’d have to take a step back into the wider drug discovery industry context.
Why now?
The second half of the 20th century was marked by some giant leaps in disease control. To name a few: mainstream antibiotics, population wide vaccination programmes, cholesterol lowering drugs, high blood pressure relief drugs, HIV antiviral therapy. However, the last couple of decades have been less buoyant. The drug discovery industry has been facing ever decreasing productivity due to low success rates in clinical trials and skyrocketing costs. Many believe the reason to be that the world has already reaped the “low hanging fruit” in medicine, namely the relatively straightforward disease-causing mechanisms which can be targeted by chemically derived (i.e. easy to manufacture) drugs which work on all patients. For example, antibiotics tackled infection and insulin tackled diabetes. What now remains is more complex diseases which require triple the effort:
1) Understand the multiple biological mechanisms which contribute to multi-faceted diseases such as Alzheimer’s or cancer;
2) Design safe medicines to target these specific mechanisms; and
3) Isolate subgroups of patients where these different mechanisms are at work so that each group can be treated separately.
New methods need to be implemented to efficiently tackle this triple challenge. The good news is that the drug discovery industry is not in this alone — the software industry has come to assist. Having made giant leaps of its own in computational power and big data science, 21st century software algorithms can assist in tackling complex disease, as long as the disease ingredients are translated into digital data points. Enter digital biology. The sequencing of the human genome in 2003 and the subsequent 100x drop in the cost of sequencing as well as the advancements in “multi-omics” research and imaging enabled the appearance of such digital data points. In the 2010s scientists came up with new tools to engineer biology, such as genome editing and AI-assisted protein design. When we add those to the mix, the field of digital biology is now not only able to analyze, but also to innovate new, synthetic solutions to the old problems!
A drive for innovation
With every patient blood sample now a data mine, the three challenges above can be tackled in silico at an unprecedented scale. The digital biology tools complement the existing suite of wet lab tools to make hypotheses, optimize experiments, and drive down the overall cost of drug discovery. Much of the innovation comes from small businesses and university spinout companies. Investment in companies which employ AI / ML techniques to study biology digitally in fields like genomics, transcriptomics, epigenetics, molecular biology has doubled since 2020 relative to the earlier period. Investments have also stayed at or above 2020 levels even in the difficult markets of 2022 and 2023.

Source: Pitchbook data
Venture investors, including my team at Mercia Ventures, have been diving into this domain. Below are some exciting, fast developing areas to watch out for under the wide umbrella of digital biology, as well as examples of how startups are trailblazing innovation in these fields.
Systems Biology
The field of systems biology aims to understand the larger picture of how the body works, bottoms up, stepping on decades of research on its individual pieces. It organizes the body into systems or layers, which are helpfully outlined below by the Institute of Systems Biology.
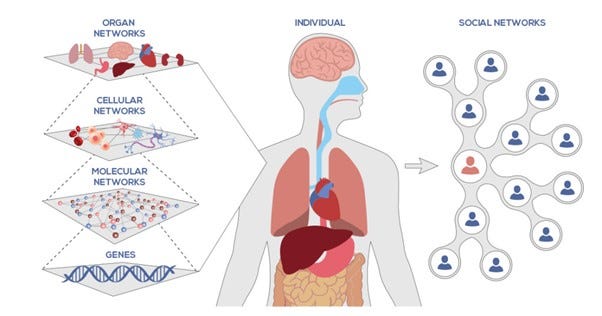
Source: Institute of Systems Biology, https://isbscience.org/about/what-is-systems-biology/
Each of the layers is a research area of its own and the lower in the hierarchy you get, the more components, or nodes, there are to analyze and model. To give an idea of the scale of this modelling challenge, the Institute for Protein Design at Washington University in the USA ran a study on protein interactions in yeast for which they modeled interactions between 8.3 million pairs of yeast proteins. Yeast contain c. 4,000–5,000 types of protein, while a human — 20,000 to 100,000 types!
Advances made by Microsoft, Google and AWS in cloud computing has resulted in unprecedented computing power becoming available to biologists so that they can study systems at such mind-boggling scale. At Mercia Ventures, we have been fortunate to work with one of trailblazers in simulating systems biology, Turbine. Based in Budapest and London, the company simulates the behaviour of cancer cells using cutting edge computational methods such as graph neural networks. Unlike other machine learning techniques which can deduct cause and effect relations between biological variables, Turbine’s approach results in interpretability or, in other words, they can answer why and how the cause resulted in the observed effect. The Turbine team is constantly vetting and adding nodes to its unified simulation engine which gets better with every experiment. Turbine has recently teamed up with synthetic biology giant Gikno Bioworks to form part of a platform of technology partners which provide different ways to assist researchers in interpreting biology and programming cells as therapeutics.
Quantum Biology
According to the Royal Society, Quantum Biology is the field of study that investigates processes in living organisms that cannot be accurately described by the classical laws of physics. This relatively new field merges developments in quantum physics into digital biology. It has already shed light on the mechanics of important processes such as photosynthesis, enzyme activity and cell metabolism.
The Mercia Ventures team has been active in this field with a recent first investment in Kuano. This Cambridge based company focuses on the enzyme as a participant in diseases’ biological pathways. Enzyme reactions are governed by complex quantum mechanical laws. To investigate them, Kuano goes beyond the atom-level chemistry that other AI-driven companies’ datasets are based on and into the electron level where the secrets of enzyme disease state behaviour are believed to be hidden. Similar to Turbine, Kuano uses a computational simulation method combined with quantum mechanics principles. The current focus of the company is to find chemical compounds that precisely bind to the enzymes they model, so that new drug candidates can be developed. The platform can be expanded beyond enzymes to other dynamic interactions in the body.
Large Language Models in biology
One cannot speak about computational methods in any field these days without noting the developments in large language models (LLMs). The potential of LLMs in digital biology can fascinate, however, there are some challenges. Unlike the vast amounts of freely available data on the world wide web that OpenAI had at its disposal when creating ChapGPT, high resolution biological data is privately held and deeply sensitive. Innovators in this field must gain access to reliable, diverse datasets, ensure data protection and security, and ensure IP rights are correctly managed between data owners and model builders.
In February 2024 a new company called Bioptimus came out of stealth mode, with a $35m seed investment check, boasting a team of experts from the biotech unicorn Owkin and Google’s DeepMind. The company declares the formidable mission of creating the “first universal foundation model in biology” so that it can understand the laws of biology. To do this, the team would need to train an AI model on all layers (or “scales” as Bioptimus calls them) of biology, from genes to cells to organ networks, as well as on the interactions between the layers. They plan to use a similar logic LLMs have used in breaking down text into tokens and structuring the relationships between them. It is no coincidence that Bioptimus refers to numerous data partnerships and a large data collaboration project called MOSAIC in its opening statement!
We at Mercia Ventures have backed multiple companies which use AI and ML techniques to make discoveries and predict outcomes within the different layers of biology: Tagomics and Wobble Genomics in the genetic layer, DxCover and Turbine in the molecular layer, Optellum in the organ layer, to name a few. I am personally so excited to see what will happen when all of these discoveries start to, figuratively speaking, talk to each other! The possibility of foundation models helping predict biological behaviours and speeding up scientific discovery is truly wonderous.
To conclude, I will leave you with one more quote which describes very well where we are today in digital biology by NYT columnist Thomas Freedman who back in 2012 wrote, “Big breakthroughs happen when what is suddenly possible meets what is desperately necessary.”